29 September 2017
9:00 - 12:30
Louvain-la-Neuve
ISBA - C115 (Seminar Room Bernoulli)
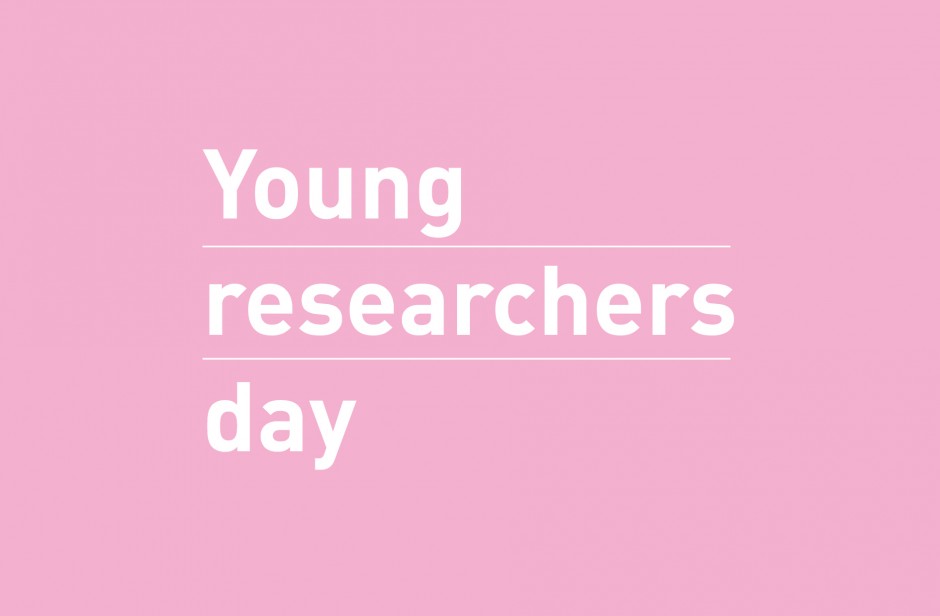
The doctoral students of ISBA give talks on the topics of their current research
9h00: Rebecca Marion
“Model Regularization for the Selection of Variable Groups in Omics Data”
Abstract:
“Model Regularization for the Selection of Variable Groups in Omics Data”
Abstract:
Statistical classification models that predict patient disease states or subtypes based on “omics” data play an important role in “personalized medicine,” making it possible to improve the quality of patient diagnosis and treatment. However, the complex structure of dependencies between variables in these “omics” data sets make it difficult to reliably identify the variables most predictive of disease. Correlated predictor variables tend to form groups that could represent a biological entity, such as a protein or metabolite, or a biological process. In such a case, it is important to select or exclude all variables in a given group so that the mechanisms of disease can be studied with greater precision and comprehensiveness. One popular approach in the literature for identifying important variables or variable groups is to impose constraints on the predictive model that induce sparsity (i.e. dependence on a reduced set of predictor variables). During this presentation, several model regularization methods of this type will be presented and compared. The empirical performance of these methods, as demonstrated in several simulation studies, will also be highlighted.
9h30: Kassu Mehari Beyene
“Time-dependent ROC curve estimation with curve fraction”
“Time-dependent ROC curve estimation with curve fraction”
Abstract:
The ROC curve and its summary measure AUC are the two commonly used tools to evaluate the classification accuracy of a continuous variable for a binary outcome. The time-dependent ROC curves have been used to assess the predictive ability of diagnostic markers for survival analysis. Several authors proposed methods to estimate the time-dependent ROC curves and AUC for a survival analysis. The validity of the estimators from these methods relay on some assumptions.
One of the assumptions is that, all subjects of the study population is susceptible to the event of interest and will eventually experience this event if the follow-up period is sufficiently long. However, this assumption may not be valid in many cases and hence studying the sensitivity of the estimators for the violation of this assumption is of substantial interest. The main aim of this article is to assess the validity of the time-dependent ROC curve and its summary measure AUC for data with cured subjects. An in depth simulations was conducted to study the performance of the estimator. The simulation studies make evident that, when the marker is known or correctly estimated, the simple method of Li et al. (2016) is insensitive to the violation of the above assumption and therefore result in valid estimates for the classification accuracy measures.
The ROC curve and its summary measure AUC are the two commonly used tools to evaluate the classification accuracy of a continuous variable for a binary outcome. The time-dependent ROC curves have been used to assess the predictive ability of diagnostic markers for survival analysis. Several authors proposed methods to estimate the time-dependent ROC curves and AUC for a survival analysis. The validity of the estimators from these methods relay on some assumptions.
One of the assumptions is that, all subjects of the study population is susceptible to the event of interest and will eventually experience this event if the follow-up period is sufficiently long. However, this assumption may not be valid in many cases and hence studying the sensitivity of the estimators for the violation of this assumption is of substantial interest. The main aim of this article is to assess the validity of the time-dependent ROC curve and its summary measure AUC for data with cured subjects. An in depth simulations was conducted to study the performance of the estimator. The simulation studies make evident that, when the marker is known or correctly estimated, the simple method of Li et al. (2016) is insensitive to the violation of the above assumption and therefore result in valid estimates for the classification accuracy measures.
10h00: Lexuri Fernandez
“Mortality modelling with Lévy processes and jumps”
“Mortality modelling with Lévy processes and jumps”
Abstract:
Human mortality shows an asymmetric mean reverting effect; it might significantly increase in a short term due to an specific occurrence, i.e. pandemic, natural catastrophe, terrorist attack, etc. but get back to the mean within a time period. However, a decrease in human mortality due to long term improvements in human life quality, such as medical or technological improvements, will remain in time. Time continuous Lévy-processes show appropriate properties to model these phenomena. Therefore, we present a stochastic mean-reverting jump-diffusion model incorporating two sided jumps for mortality modeling.
10h30: Coffee Break
11h00: Stefka Asenova
“Graphical models and extremes”
Abstract:
Graphical models form a class of statistical models designed for analyzing ensembles of stochastic variables whose joint law is determined by a set of conditional independence relations. A graph is an Independency map of the joint probability law if graphical separation implies conditional independence. Such structures, commonly known in literature, are Markov fields and Bayesian networks. When interest is in extreme values of the variables the analysis must include both the theory of Multivariate Extremes and Graphical models.
Extreme value theory is the branch of probability theory and statistics which aims at providing models for rare events and for observations that occur with low frequency but have a potential high impact, i.e., for the tails of statistical distributions. Examples include high water levels leading to flooding, financial market turmoil, and catastrophe insurance claims. Of noteworthy importance is the issue of tail dependence, that is, the propensity of such extreme values to occur simultaneously in many variables at once.
The aim of the project is then to develop models for extreme values of random variables whose dependence structure can be represented by a graph via conditional independence relations.
The current research focuses on tree structures. The goal is to combine the theoretical results about asymptotic convergence of a tree graphical model and one of the novel estimators of multivariate tail dependence to obtain an estimator of extreme dependencies in a regularly varying tree model. The scope of the paper is to illustrate how both theories can be combined to draw inference on the tail dependence in case a tree structure is known or assumed.
Graphical models form a class of statistical models designed for analyzing ensembles of stochastic variables whose joint law is determined by a set of conditional independence relations. A graph is an Independency map of the joint probability law if graphical separation implies conditional independence. Such structures, commonly known in literature, are Markov fields and Bayesian networks. When interest is in extreme values of the variables the analysis must include both the theory of Multivariate Extremes and Graphical models.
Extreme value theory is the branch of probability theory and statistics which aims at providing models for rare events and for observations that occur with low frequency but have a potential high impact, i.e., for the tails of statistical distributions. Examples include high water levels leading to flooding, financial market turmoil, and catastrophe insurance claims. Of noteworthy importance is the issue of tail dependence, that is, the propensity of such extreme values to occur simultaneously in many variables at once.
The aim of the project is then to develop models for extreme values of random variables whose dependence structure can be represented by a graph via conditional independence relations.
The current research focuses on tree structures. The goal is to combine the theoretical results about asymptotic convergence of a tree graphical model and one of the novel estimators of multivariate tail dependence to obtain an estimator of extreme dependencies in a regularly varying tree model. The scope of the paper is to illustrate how both theories can be combined to draw inference on the tail dependence in case a tree structure is known or assumed.
11h30: Dimitra Kyriakopoulou
“Exponential-type GARCH models with linear-in-variance risk premium”
“Exponential-type GARCH models with linear-in-variance risk premium”
Abstract:
One of the implications of the intertemporal capital asset pricing model (CAPM) is that the risk premium of the market portfolio is a linear function of its variance. Yet, estimation theory of classical GARCH-in-mean models with linear-in-variance risk premium requires strong assumptions and is incomplete. We show that exponential-type GARCH models such as EGARCH or Log-GARCH are more natural in dealing with linear-in-variance risk premia. For the popular and more difficult case of EGARCH-in-mean, we derive conditions for the existence of a unique stationary and ergodic solution and invertibility following a stochastic recurrence equation approach. We then show consistency and asymptotic normality of the quasi maximum likelihood estimator under weak moment assumptions. An empirical application estimates the dynamic risk premia of a variety of stock indices using both EGARCH-M and Log-GARCH-M models.
12h00: Hervé Azabou
“Accelerated Failure Time Model with smoothed error distribution and one censored covariate using EM algorithm"
“Accelerated Failure Time Model with smoothed error distribution and one censored covariate using EM algorithm"
Abstract:
Most of the existing parametric and semiparametric methods in survival analysis assume that covariates are fully observed. However, in some studies, covariates are incomplete (censored or missing). For example, measurement below a detection limit often happens when measuring concentration in blood sample or when trying to detect pollution in environmental studies.
Some methods are commonly used to handle censored covariates in survival analysis. One has (i) Single imputation, or the substitution method consisting of replacing each unobserved or censored value by a fixed value and then applying a standard statistical technique to obtain the estimates. This method is not satisfactory and does not have theoretical justification. (ii) Complete case method, consisting of removing all entries in the database having at least one censored value for any covariate to be used in the analysis. (iii) Multiple imputation and data augmentation consisting of replacing each censored value by a set of m > 1 plausible values reflecting the uncertainty about the values to impute. In that way, One obtains m datasets on which standard techniques are applied. And (iv) the maximum likelihood based approaches. Here, the censoring pattern of the covariates is taken into account for the construction and maximization of the likelihood to reflect the uncertainty due to censoring.
We propose a method based on EM algorithm (Dempster et al., 1977), to estimate and make inference in the context of semiparametric accelerated failure time (AFT) models where both time-to-event variable and one covariate are subject to censoring. Although the method is exposed for the right censoring case, it can be extended to handle left and interval censoring in both time-to-event variable and covariate. We use a smoothed error distribution to ensure the flexibility. This is done by using the idea underlying P-splines (Penalized B-splines), but with each B-spline in the basis approximated by a Gaussian density, the main advantageous being that it can capture all error distributions with zero mean and unit variance. That approximation was first proposed by Komárek et al. (2005).
The standard errors are obtained using the method proposed by Oakes (1999) which is 1 one method among others to compute standard errors in the context of EM algorithm. We performed simulations to assess the performance of the method and compare it to the complete case method. It appears that the proposed and the CC methods give unbiased estimators, but the proposed method performs better in terms of mean squared error.
Some methods are commonly used to handle censored covariates in survival analysis. One has (i) Single imputation, or the substitution method consisting of replacing each unobserved or censored value by a fixed value and then applying a standard statistical technique to obtain the estimates. This method is not satisfactory and does not have theoretical justification. (ii) Complete case method, consisting of removing all entries in the database having at least one censored value for any covariate to be used in the analysis. (iii) Multiple imputation and data augmentation consisting of replacing each censored value by a set of m > 1 plausible values reflecting the uncertainty about the values to impute. In that way, One obtains m datasets on which standard techniques are applied. And (iv) the maximum likelihood based approaches. Here, the censoring pattern of the covariates is taken into account for the construction and maximization of the likelihood to reflect the uncertainty due to censoring.
We propose a method based on EM algorithm (Dempster et al., 1977), to estimate and make inference in the context of semiparametric accelerated failure time (AFT) models where both time-to-event variable and one covariate are subject to censoring. Although the method is exposed for the right censoring case, it can be extended to handle left and interval censoring in both time-to-event variable and covariate. We use a smoothed error distribution to ensure the flexibility. This is done by using the idea underlying P-splines (Penalized B-splines), but with each B-spline in the basis approximated by a Gaussian density, the main advantageous being that it can capture all error distributions with zero mean and unit variance. That approximation was first proposed by Komárek et al. (2005).
The standard errors are obtained using the method proposed by Oakes (1999) which is 1 one method among others to compute standard errors in the context of EM algorithm. We performed simulations to assess the performance of the method and compare it to the complete case method. It appears that the proposed and the CC methods give unbiased estimators, but the proposed method performs better in terms of mean squared error.
12h30: Sandwich lunch in the cafeteria
Categories Events: