15 May 2018
4:30 PM
CORE, b-135
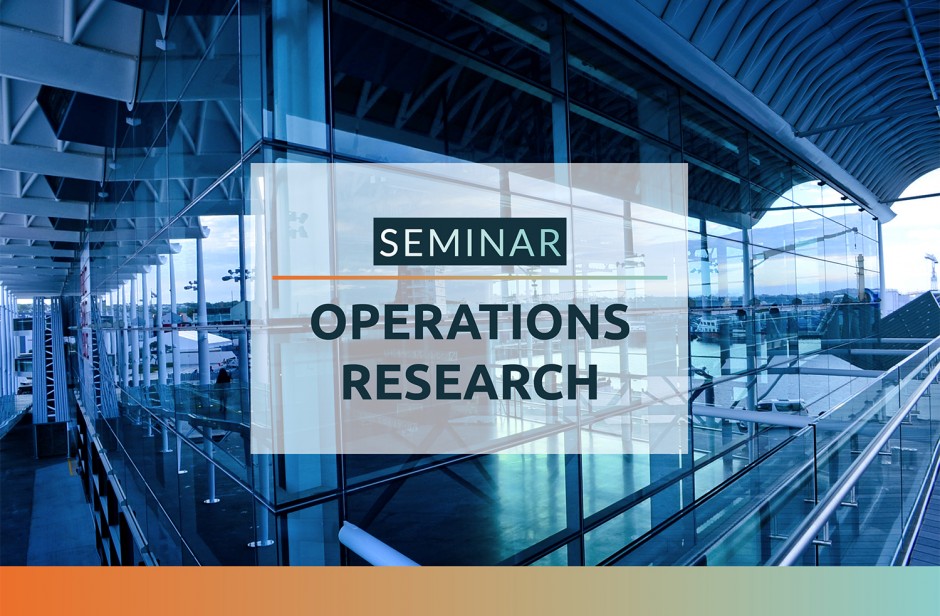
Automated design of first-order optimization methods
Adrien Taylor, Centre de recherche INRIA de Paris
In this talk, we will describe a novel constructive technique for devising efficient first-order methods for a wide range of large-scale convex minimization settings, including smooth, non-smooth, and strongly convex minimization.
The design technique takes an "ideal method", performing a series of subspace-searches, and constructs a family of methods sharing the same worst-case guarantees.
We show that this technique yields optimal methods in the smooth and non-smooth cases and derive new methods for these cases, including methods that forego knowledge of the problem parameters, at the cost of a one-dimensional line search per iteration.
In the strongly convex case, we show how numerical tools can be used to perform the construction, and show that resulting method offers an improved convergence rate compared to Nesterov's celebrated fast gradient method.
This talk is mainly based on recent results obtained with Yoel Drori (see arXiv). The introductory part is based on joint works with Julien Hendrickx, François Glineur, and Etienne de Klerk.