December 05, 2017
4:30 PM
CORE, room b-135
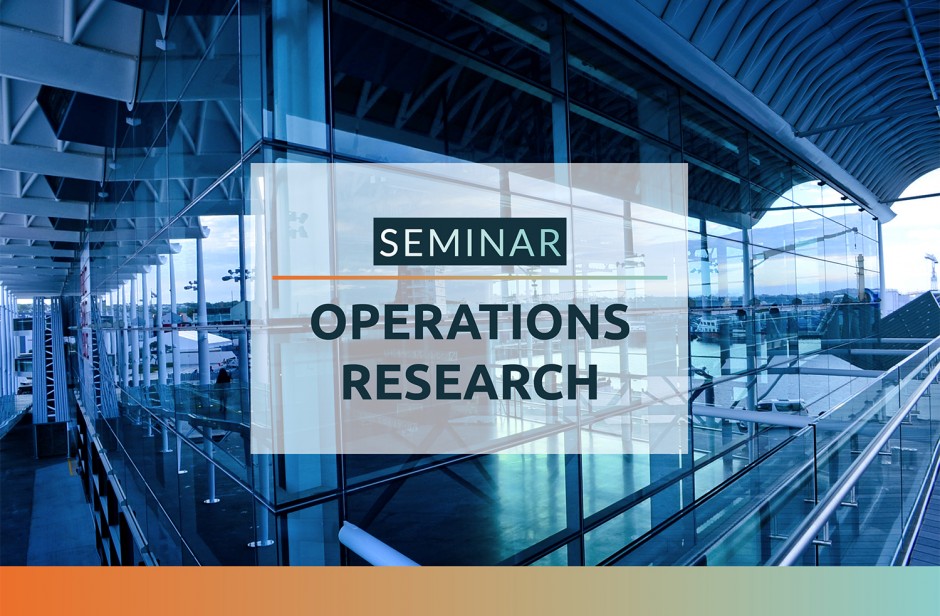
Model Uncertainty and Distributional Robustness in Stochastic Optimization
Georg Pflug, Universität Wien
Decision making in a stochastic environment requires the formulation of a stochastic model (e.g. for prices, demands, weather and similar risks). Since these models are mostly based on observed data, statistics tells us that possibly several models are compatible with the observations, and if we choose one of them, there is the risk of having chosen a wrong one (i.e. the model risk).
Model ambiguity means that a set of models is chosen and distributionally robust decisions are those which are good (but not optimal) for all of the candidate models. Mathematically speaking, we
look for minimax solutions and saddle points. We review some results in this direction and define the costs for ambiguity and the reward for distributionally robust solutions.
We use examples from portfolio selection, from insurance design and from hydrostorage management. In addition, we show how option prices based on an acceptability criterion depend on the degree of model ambiguity.